
If it were possible to eliminate all the errors in the world, take into account all circumstances and predict all consequences, then science would become unnecessary, and life would be boring and repetitive. Without mistakes, there would be no evolution or diversity. We would either be at a standstill, or completely extinct.
Let’s imagine a tall, shallow glass case. Along its narrow base, there is a row of several small, equal-sized compartments. Above the compartments are rows of wooden pegs driven into the back of the case in a pattern of repeating quincunxes––four pegs making up the corners of a square, and a fifth in its center––so that each row is offset from the neighboring rows by half the distance between the pegs. The front of the case is covered with a glass pane, the sides are wooden panels. At the top, in the very center, there is a small hole with a protruding funnel.
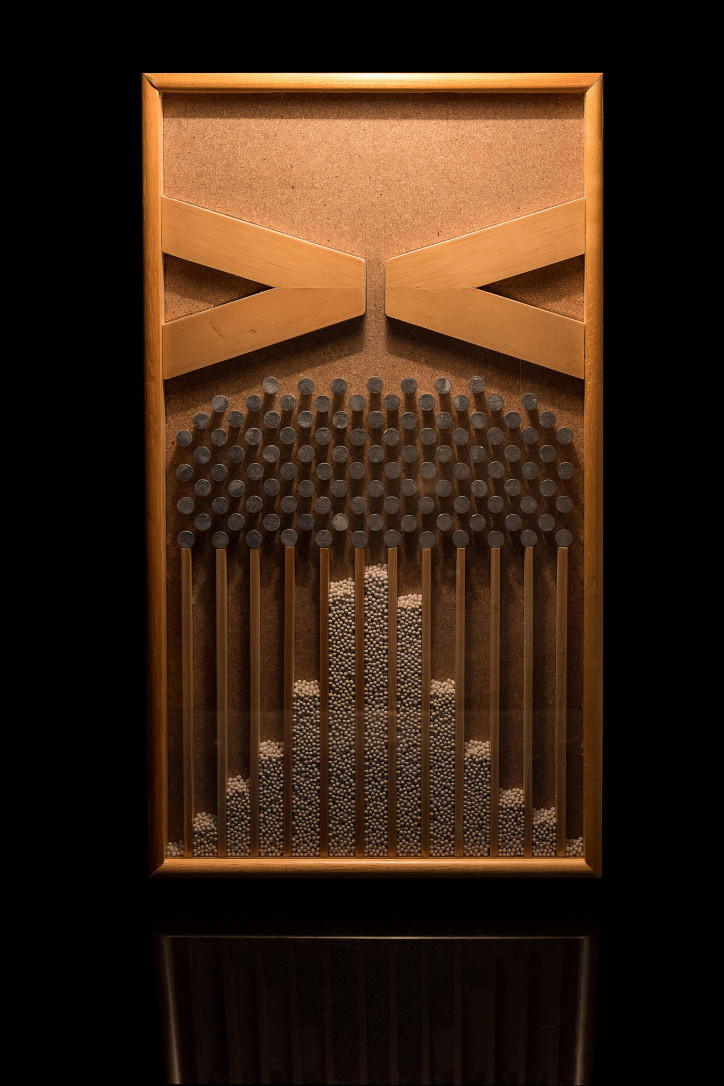
This device is called a “bean machine” and requires a handful of beans to be poured into the display case through the hole at the top. Instead, let’s imagine tipping in a large quantity of metal balls. A shining stream pours from the funnel, the balls hit the first wooden pegs––and thus begins a phenomenon that is hard to explain. The balls are quickly distributed throughout the case, clattering as they hit the wood. It’s absolute chaos, the balls striking the pegs at random, appearing to leap from one side of this strange device to the other. When the balls reach the bottom, they land in one of the compartments. But they’re not distributed randomly, as one